Future large-scale cell culture platform: from "multi-scale" to "intelligent"
- Share
- Issue Time
- Dec 2,2024
Summary
Future large-scale cell culture platform: from "multi-scale" to "intelligent"
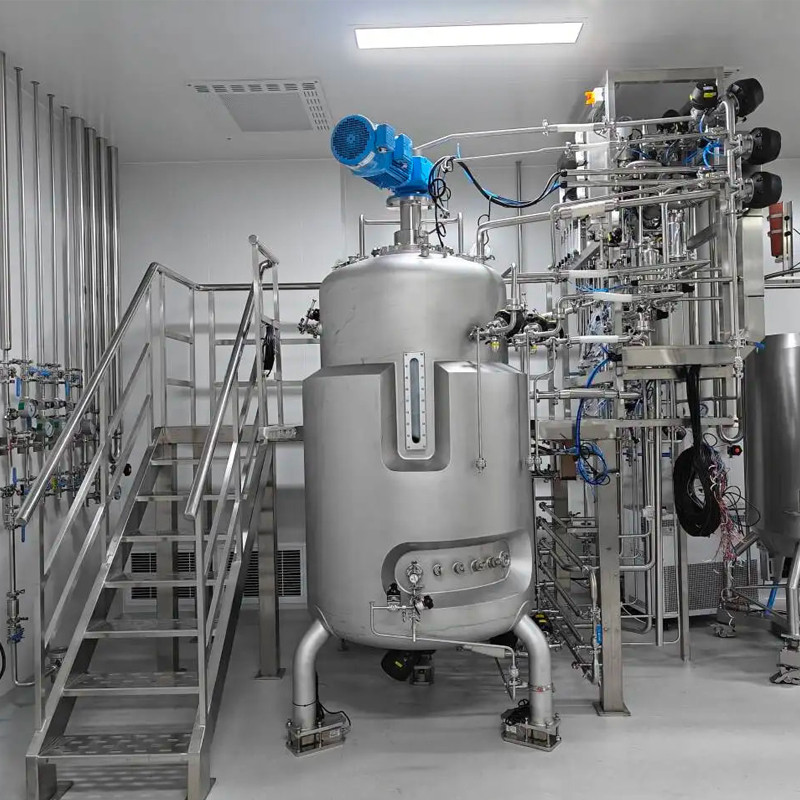
For decades, mammalian cells have been used as carriers for producing therapeutic proteins. Therapeutic proteins are widely used in the diagnosis and treatment of diseases, forming a huge market. The global biopharmaceutical market was valued at $237.2 billion in 2018 and is expected to reach $389 billion by 2024, with a compound annual growth rate of 8.59% during the forecast period from 2019 to 2024.
Between 2006 and 2010, approximately 55% of biopharmaceuticals were expressed in mammalian cells, and between 2010 and 2014, 60% of recombinant therapeutic proteins were also based on mammalian cell expression.
Among them, monoclonal antibody drugs have the largest number, with a global monoclonal antibody market size of around 164 billion US dollars in 2019, accounting for over 50% of the global biopharmaceutical market share, making it the largest sub industry in the global biopharmaceutical industry.
Therefore, the increasing demand for protein products produced through mammalian cells has made the research and development of large-scale animal cell culture technology an important task in the field of biopharmaceuticals.
In the past 20 years, the biomanufacturing industry has made significant improvements in process control. Computer online control is mainly used in the stirred bioreactor, equipped with online sensors such as pH electrode, dissolved oxygen electrode, exhaust gas mass spectrometer, live cell sensor, etc., to achieve the collection of multiple parameters such as live cell count, concentration of extracellular metabolites such as glucose, lactate, ammonia, and glutamine.
With the increasing maturity of PAT process control technology, the combination of online sensors with system models of cellular physiological states, and the introduction of omics technology, can achieve data-driven guided process control and ensure product quality by establishing process data processing methods, classifying and analyzing data in depth.
After years of research, Zhang Siliang and others proposed a theory based on multi-scale parameter analysis of genes, cells, and reactors. The complex biological processes in bioreactors are divided into gene scale, cell scale, and bioreactor scale, with information flow, material flow, and energy flow between different scales.
By studying the nonlinear relationship between them and their impact on the entire system, we can identify methods to optimize and amplify the solution process.
In large-scale animal cell culture, the biological reaction process of living cells has complex physiological and metabolic characteristics, so it is crucial to conduct multi-scale parameter analysis of the cell culture process in bioreactors.
However, there are still some limitations to the application of this theory in the era of big data. In the actual production process, it is extremely difficult to process the massive data generated during multi-scale parameter analysis of cells and various sensor data obtained during the reaction process.
Finding key causal relationships in these data and proposing corresponding process optimization strategies is a time-consuming and laborious task for manual processing. Therefore, it is necessary to apply machine learning to the analysis and decision-making of big data in biological processes.
In the face of large-scale and diversified cultivation of animal cell lines, various new animal cell reactors can be developed, personalized and parallel cultivation strategies can be formulated, and intelligent control of the cultivation process can be achieved. Bioreactor coupled online sensors, especially spectral sensors such as online Raman analyzers, online mid infrared analyzers, online fluorescence analyzers, etc., are gradually being applied in industrial process analysis.
In the implementation of multi-scale biological process optimization, online Raman spectroscopy, online fluorescence spectroscopy, and online mid infrared spectroscopy will generate a large amount of data, especially online data containing a lot of process related information.
In the future, literature data and omics data can be used to form a knowledge graph spectrum, and machine deep learning can be used to guide the cell culture process.
In 2018, the "fully automated stem cell induction and cultivation equipment" was successfully developed, achieving for the first time the automation of cell induction based on machine learning and artificial intelligence algorithms.
Equipment technology was established for cell culture, microscopic online observation, liquid transfer, algorithm recognition, clone picking, and device control, realizing the automation of induction and cultivation, amplification, imaging, downstream differentiation, and other functions of multi-functional stem cells.
While reducing the production cost of stem cells, the quality of cell preparation was improved, laying the foundation for the production of large-scale biological products. The era of big data has arrived, and intelligent biomanufacturing based on big data is in line with the trend of biomanufacturing industry development.
With the continuous development of biosensing technology, more and more biological process data can be obtained. By using artificial intelligence to process data and extract relationships between information flows at different scales, true intelligent biomanufacturing can be achieved.