How to make software "understand" fermentation metabolism?
- Share
- Issue Time
- Feb 18,2025
Summary
How to enable fermentation control software to dynamically analyze metabolic pathways, achieve intelligent control from "experience driven" to "model predictive driven", and significantly improve the efficiency and stability of the fermentation process?
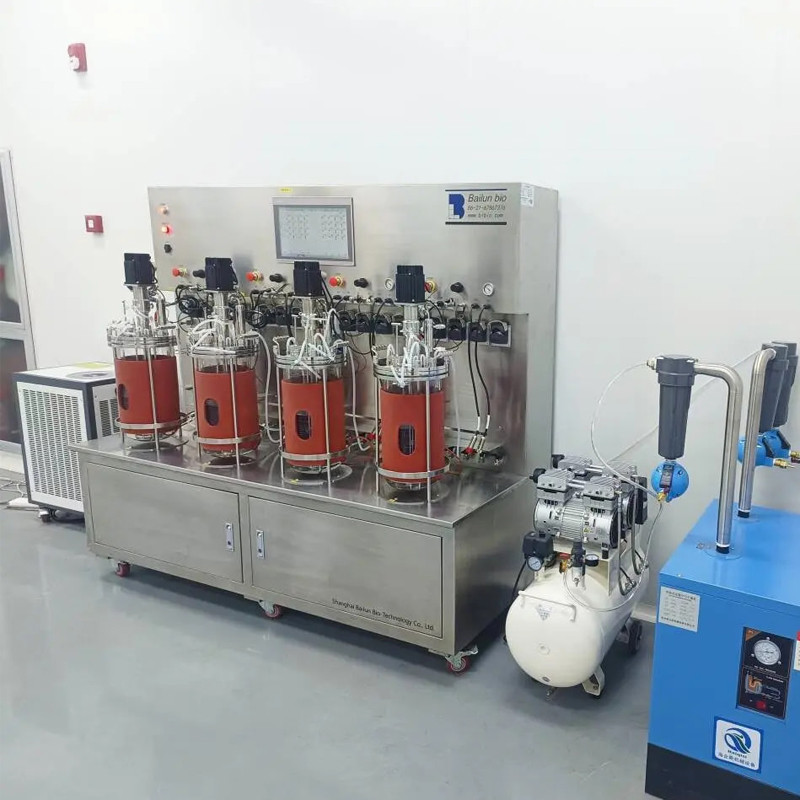
Step 1: Data Collection - Creating a Digital Portrait of Fermentation
The first step in making software "understand" the fermentation process is to collect a large amount of key data.
These data include parameters such as temperature, pH value, dissolved oxygen level, stirring rate, and substrate concentration during the fermentation process, as well as monitoring metabolic data such as metabolite concentrations (such as glucose, lactate, amino acids), enzyme activity, and ATP/NADH levels.
In addition, researchers will integrate genomic, transcriptomic, proteomic, and metabolomic data to comprehensively analyze the metabolic potential of microorganisms.
Through sensors and online monitoring devices, this data can be transmitted in real-time to software, providing a solid foundation for subsequent analysis.
The temperature difference of 1 ℃ in the bioreactor has caused the enterprise to lose millions?
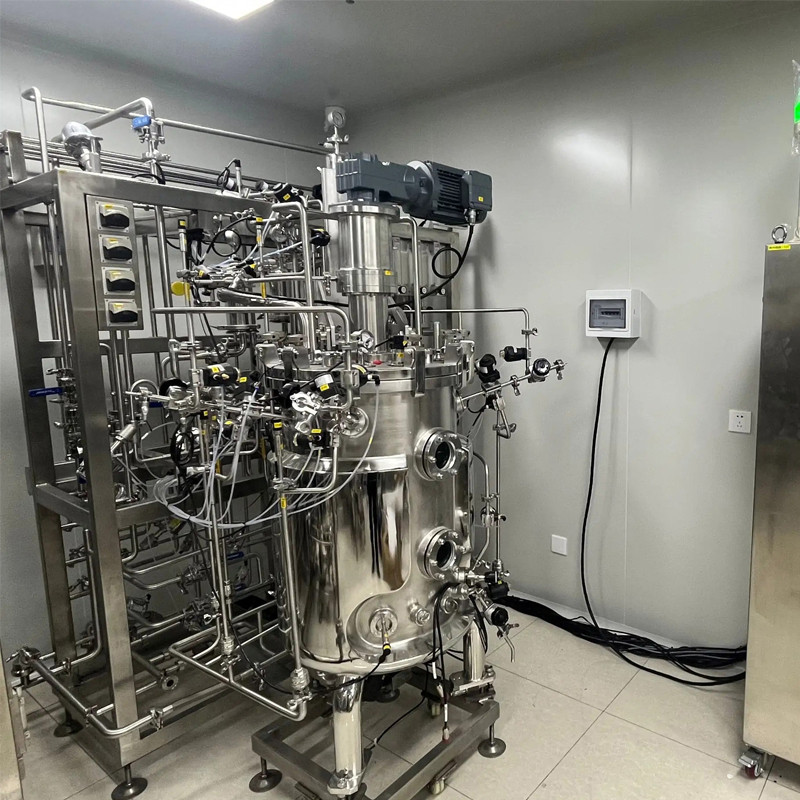
Step 2: Metabolic Modeling - From Data to Model
After obtaining these data, the next step is to construct a metabolic network model.
Researchers extract the metabolic pathways of target strains from genomic data and convert them into mathematical models using specialized tools such as COBRApy and metaFlux.
Through time series data such as substrate consumption rate and product generation rate, the model can infer changes in metabolic flux, identify rate limiting steps and key metabolic nodes.
According to the requirements, researchers can choose different types of dynamic models, flux balance analysis models, machine learning models, and even hybrid models to provide accurate prediction and control strategies for the fermentation process.
On the fourth day of the Lunar New Year, I believe DeepSeek will help promote the intelligence of biomanufacturing!
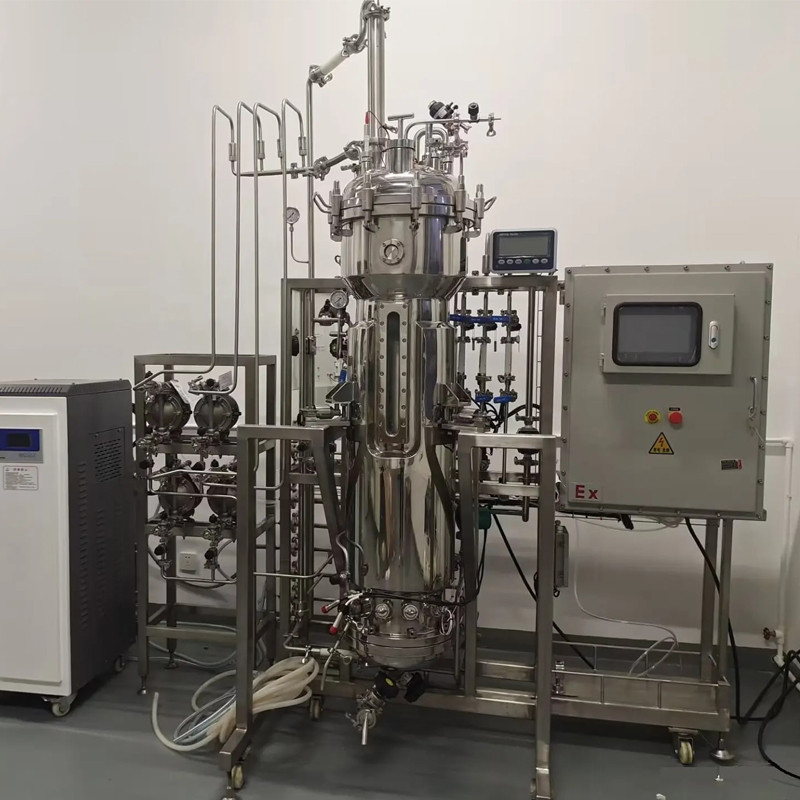
Step 3: Software Integration
Integrating metabolic models with fermentation control software is a crucial step towards achieving intelligence.
Through OPC-UA or API technology, sensor data can be input into the model in real-time, and the software dynamically adjusts fermentation parameters based on model predictions.
At the same time, by combining feedforward control and feedback control strategies, the software can predict and optimize the fermentation process in advance and in real time.
In addition, the design of a visual interface is also crucial as it can help operators intuitively understand the fermentation process and software control strategies, thereby improving operational efficiency.
Big model+big data+knowledge graph=the future of intelligent biological fermentation?
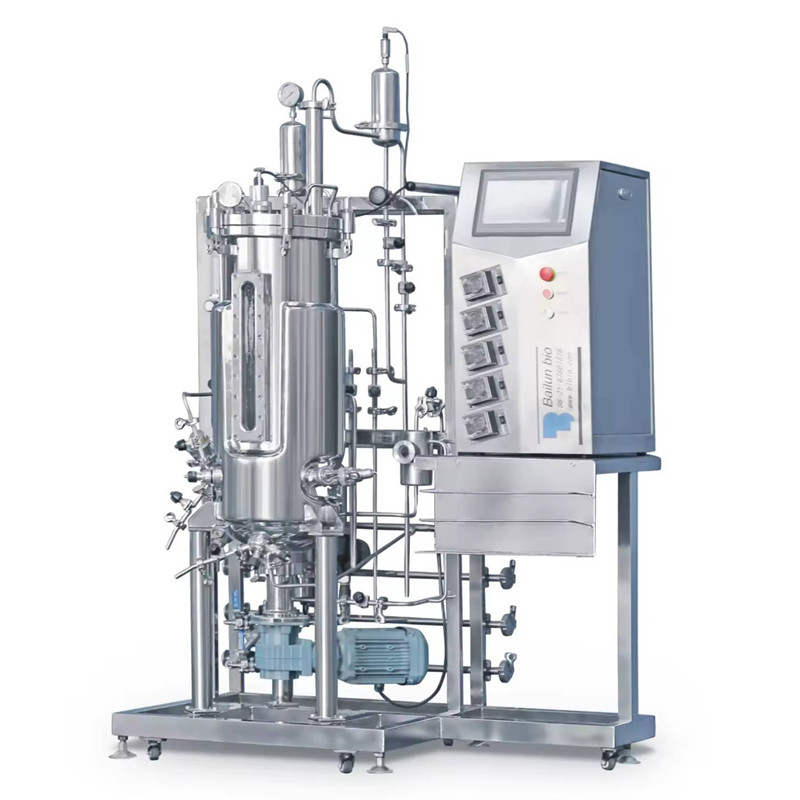
Step 4: Model Validation and Optimization
Researchers evaluated the reliability and accuracy of the model through offline simulation and small-scale fermentation tank testing.
According to experimental data, model parameters can be further calibrated, secondary metabolic pathways can be simplified, and even reinforcement learning algorithms can be embedded to achieve adaptive optimization.
Taking yeast production of ethanol as an example, through real-time monitoring and model prediction, ethanol production increased by 15% and fermentation cycle shortened by 10%.